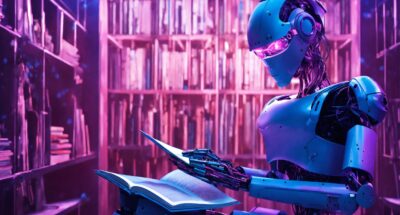
Six books on AI written by intelligent humans
The old-fashioned printed word offers valuable insight on the latest technology. Here is our guide....
by Tomoko Yokoi Published 4 April 2024 in Artificial Intelligence • 11 min read
Recent findings reveal that most CEOs recognize the urgency of adopting GenAI to maintain a competitive edge, perceiving significant potential to uncover new insights, enhance operational efficiency, and strengthen risk management. Yet this sense of urgency is tempered by uncertainty due to its nascent nature, which brings questions about its capabilities, ethical use, and long-term societal implications. Business leaders, as we learned from a recent CEO Roundtable gathering at IMD, are seeking clarity on how to use GenAI effectively and safely, especially when there are still many questions surrounding the technology and its providers.
This article provides a roadmap for integrating GenAI into your organization responsibly and effectively. It begins by outlining key considerations for utilizing GenAI. Drawing on previous research into the hallmarks of successful digital organizations, it identifies essential qualities for evolving into a GenAI-centric organization: adopting a forward-thinking mindset towards generative technologies, cultivating a workforce proficient in AI, prioritizing data integrity, and fostering a culture of collaborative intelligence.
“By not advancing, organizations risk falling behind. It is imperative to act and innovate.”
Given the novelty of GenAI, organizations are engaging in experimentation and exploration. Participants at our recent CEO Roundtable indicated a primary focus on experimenting with internal use cases, such as knowledge management and back-office support. This is reflective of a broader trend where more than half of the respondents to a VentureBeat survey said their organizations are experimenting with AI, but that only 18% of those companies have begun implementing it.
When it comes to new technologies, leaders often adopt a strategy of placing many small bets, rather than a handful of large ones. This approach allows them to experiment on multiple fronts, scale up successes quickly, and phase out unsuccessful ones with minimal losses. This method is particularly relevant given the current reliability concerns with GenAI. Its tendency to “hallucinate” or produce inaccurate or unexpected results, coupled with its opaque decision-making process, has led to caution in its deployment of customer-facing applications.
However, this cautious approach should not lead to inaction. By not advancing, organizations risk falling behind. It is imperative to act and innovate. Organizations need to explore ways to leverage GenAI not just within their operations, but also to optimize how they engage and serve customers. Moreover, the potential impact of GenAI on the future organizational structure and the employees’ learning calls for new organizational designs.
Companies across diverse sectors are making significant investments in AI and investigating how to scale the technology across their organizations. How can organizations strategically integrate GenAI for long-term success? To initiate this journey, I suggest a three-step approach. (Figure 1).
First, it is important to ensure that your organization possesses the characteristics essential to operate as a successful digital entity. Based on prior research, my colleagues and I have identified these as the ability to rapidly adapt and self-organize, delivering value through emerging technologies. We highlighted four key attributes: a digital-first mentality, a workforce skilled in digital technology, a commitment to data-informed decision-making, and the ability to self-organize and scalable work orchestration.
A digital-first mindset is about prioritizing digital solutions over traditional, process-oriented methods. Excelling at open innovation, building hyperawareness, and becoming an agile organization are some ways to build a digital-first mindset. Equipping staff with the necessary skills to navigate and utilize new technologies and processes is crucial for building a digitally proficient workforce. Such organizations are adept at using tools and data to deploy and reconfigure both human and capital resources, with decision-making driven by data rather than intuition. Additionally, these organizations excel in cultivating a culture of collaborative learning, encouraging idea-sharing and problem-solving across diverse functions and groups. Leaders help by setting clear goals, encouraging boundary-spanning collaboration, providing liberal access to relevant information, and trusting their employees to bring their best expertise to bear for each challenge.
As GenAI continues to evolve, the importance of these characteristics becomes even more pronounced. It is crucial that organizations not only preserve these qualities but also enhance and adapt them to align with the novel challenges and opportunities that come with the progress of GenAI. To navigate this evolution successfully, the next step involves developing a comprehensive understanding of GenAI’s distinct characteristics.
These models, like all AI, are prone to errors. Given the broad and general application of these systems, organizations need to implement robust guardrails – safeguards and protocols – to mitigate risks and ensure reliable performance for both developers and users. Take the example of software company Salesforce, which established a new framework to guide the responsible use of AI. These guidelines encompass five key areas: accuracy, which involves accounting for verifiable results and communicating uncertainties, safety, which includes efforts to minimize bias and protect privacy, honesty, which requires transparency about data usage and AI-generated content, empowerment, which seeks to keep humans involved in decision-making, and sustainability, which advocates for developing optimally-sized models to minimize environmental impact.
Another significant aspect is the risk of GenAI models inadvertently exposing sensitive training data. This is particularly problematic if the data includes proprietary or copyrighted content. Incidents such as Samsung employees inadvertently sharing protected source code on ChatGPT, along with lawsuits against various companies for alleged copyright infringements, have elicited a range of reactions from the corporate sector. While corporations such as Samsung, Verizon, and Apple, as well as financial giants such as Deutsche Bank and JP Morgan Chase, have opted to ban or limit generative AI tools in the workplace, others have taken a different path. Some organizations are developing bespoke AI systems that leverage internal data and are deployed exclusively within their IT environments. The decision to use such technology depends on the specific needs and risk profile of each business.
Building upon these insights, organizations should conduct an in-depth analysis to pinpoint where GenAI can add the most value. This involves assessing current processes, identifying potential applications, and determining how GenAI can solve existing problems or enhance performance. Mastercard, the global payments processing firm, put together an internal council of AI-informed leaders from all areas of the business to evaluate GenAI use cases in areas such as fraud detection, internal knowledge management, and personalization. By undertaking such an analysis, organizations can create a targeted strategy for where and how to deploy GenAI effectively. By following these three steps, organizations can lay a solid foundation for becoming a GenAI organization.
For organizations lacking an established AI governance framework, embracing the four key traits of digital-first organizations – adapted to the unique challenges and opportunities of GenAI – may serve as a helpful guide for successful integration. I outline these four adapted traits below (Figure 2).
Generative-forward mindset: This mindset is about acknowledging the transformative potential of GenAI beyond mere automation to encompass all business areas, including content creation and customer engagement. Leadership plays a pivotal role here, where acting as an advocate is essential. The rapidly evolving landscape requires leaders to develop a vision that extends beyond current metrics and ROI forecasts. It often demands a “leap of faith” approach.
Embracing this uncertainty will be essential, and leaders should encourage their executives to also delve into the data and explore the possibilities of GenAI. They should also be prepared to redeploy human resources in more value-adding ways that reconfigure the business model for greater efficiency and productivity, while more repetitive core processes can be automated.
While this may be initially disconcerting for some employees, there are examples of GenAI driving job creation and even creating new career paths. US-based Prolific, for example, has developed a new value proposition, connecting AI developers with research participants who help to review AI-generated material for inaccuracies or potential to do outright harm. This process has given rise to new categories of “AI workers.” Amongst these are data annotators, who scrutinize the data prior to its integration into AI systems and assess the generated results; and prompt engineers, who are tasked with training AI models to deliver accurate and relevant responses to questions real people are likely to pose. These roles are becoming increasingly crucial and are something that organizations should consider incorporating into their workforce.
AI fluent workforce: This quality extends beyond digital competence to a deeper fluency in AI and GenAI. It underscores the importance of having a workforce skilled in using, understanding, and ethically managing GenAI systems. Many organizations have placed an emphasis on AI education, particularly with the introduction of ChatGPT, highlighting the necessity of equipping the workforce with the knowledge to navigate more complex AI technologies capable of dynamic interaction. In the past ten months, global leaders such as PwC, Deloitte, EY, and KPMG have all pledged substantial investments into training their employees on GenAI technologies.
Developing an AI fluent workforce involves advancing from basic AI literacy to offering targeted training on the capabilities, limitations, and ethical considerations of AI. Leadership is key here, with a focus on cultivating AI literacy amongst senior executives. For example, Mastercard actively engaged in educating their senior executives and board members about generative AI, covering its capabilities, regulatory requirements, and strategies for its implementation, Once equipped with this knowledge, leaders are in a better position to advocate for the development of shared infrastructures and foster the use of these technologies across various functions and departments.
“Data integrity” focus: At the foundation of a robust AI framework lies the imperative to mitigate bias, safeguard privacy, and respect intellectual property rights. With the advent of GenAI, the risk of inadvertently revealing sensitive training data becomes a pressing concern. This underscores the need for a pre-emptive focus on the implications of bias, discrimination, and decision-making with non-verified data. This requires interdisciplinary collaboration, bringing together expertise from data privacy, customer services, legal, marketing, and development to identify and mitigate risks.
Given the particularly sensitive nature of data within the insurance sector, Swiss insurer Die Mobiliar proactively formulated a data strategy well before the emergence of ChatGPT. It assembled an interdisciplinary team from compliance, business security, data science, and IT architecture. This team’s mission is to ensure that its data strategy and ethical considerations are aligned. They accomplish this by exchanging knowledge, offering advice, and maintaining a comprehensive perspective. The team is governed by a board represented by wider stakeholders who carry information back to their respective business lines and alert the team to relevant issues. This two-tiered governance model provides direction and encourages cooperative efforts on matters of digital responsibility, establishing a solid foundation for the use of GenAI that is committed to data integrity.
Collaborative intelligence: GenAI experimentation is not an isolated pursuit; it’s a collaborative effort that benefits from shared insights and advancements. The learnings from one department can propel innovation throughout an organization, emphasizing that the value lies not just in individual achievements but in how they collectively uplift the whole. This can be done by building what transformation experts describe as corporate centers of excellence (COEs), that is, a model that incorporates a centralized knowledge base to create hardwired processes and standardized systems that efficiently produce and deploy AI models and solutions across the organization. These COEs can be standalone cost centers that are either fully funded by corporate headquarters or cofounded by various business units. Alternatively, it can operate under IT as a separate unit with clear boundaries or under a dominant business unit or key function such as marketing.
GenAI also introduces a dynamic that requires continual interaction with technology, moving beyond static learning curves. As these tools learn autonomously, employees face the ongoing challenge of adapting to their evolving capabilities. The integration of GenAI into the workplace is redefining the interplay between human cognition and artificial intelligence, raising critical questions about how to employ it in a way that enhances rather than hinders human capabilities. To effectively navigate this, it is useful to first differentiate among the participants in the interaction – whether they are individuals, groups, or machines – and to identify the initiator of the interaction, be it a human or a machine. This categorization results in six possible collaborative uses of generative AI, such as a “CoachGPT”, which provides employees with suggestions on managing their work by observing what they do and their environment; or “BossGPT,” which advises and coordinates a group of people on what they could or should do to maximize team output. GenAI is becoming a core component of our cognitive processes, demanding a thoughtful approach to its adoption that prioritizes augmentation and seamless integration into the fabric of organizational intelligence.
So, it’s now time to ask yourself:
– How far do you think your organization is from having a generative-forward mindset?
– How AI fluent is your workforce? Are you thinking about how AI can augment the “value-add” areas within your organization?
– Is your approach to maintaining data integrity robust enough to ensure the reliability of the data underlying your decision-making process?
– What new organizational designs could you envision that would empower your employees to effectively leverage the capabilities of GenAI?
To deliver on the promise of generative AI, leaders must act both as advocates and stewards. As advocates, leaders champion the interconnected use of these technologies across departments, encourage digital literacy amongst senior executives, and mobilize the organization to explore the potential. As stewards, they are responsible for the conscientious deployment of these technologies, prioritizing stakeholder trust, and addressing ethical considerations.
In this dual role, leaders must balance the drive for technological advancement with the duty of safeguarding their organization’s ethical standards and public trust. Most importantly, leaders must ensure that their advocacy for GenAI does not neglect the workforce, but instead prepares and empowers employees for the evolving job landscape. By doing so, they mitigate the risk of technology-induced job displacement, underscoring the notion that it is strategic decision-making, rather than technology itself, which truly shapes the future of work.
Researcher, Global Center for Digital Business Transformation, IMD
Tomoko Yokoi is an IMD researcher and senior business executive with expertise in digital business transformations, women in tech, and digital innovation. With 20 years of experience in B2B and B2C industries, her insights are regularly published in outlets such as Forbes and MIT Sloan Management Review.
24 hours ago • by Öykü Işık in Artificial Intelligence
The old-fashioned printed word offers valuable insight on the latest technology. Here is our guide....
29 April 2024 • by Jim Pulcrano in Artificial Intelligence
A flurry of venture capital activity presents investors with a challenge: how to distinguish genuine innovators from opportunistic players capitalizing on the AI hype. Enter Harshul Sanghi, a seasoned technology investor with...
26 April 2024 • by José Parra Moyano in Artificial Intelligence
AI holds incredible promise to improve virtually every aspect of our lives, but we can't ignore its risks, mishaps, and misuses, author Art Kleiner tells José Parra Moyano....
23 April 2024 • by Jerry Davis in Artificial Intelligence
GenAI can deliver cost and productivity benefits for colleges and students, but there is a dark side....
Explore first person business intelligence from top minds curated for a global executive audience