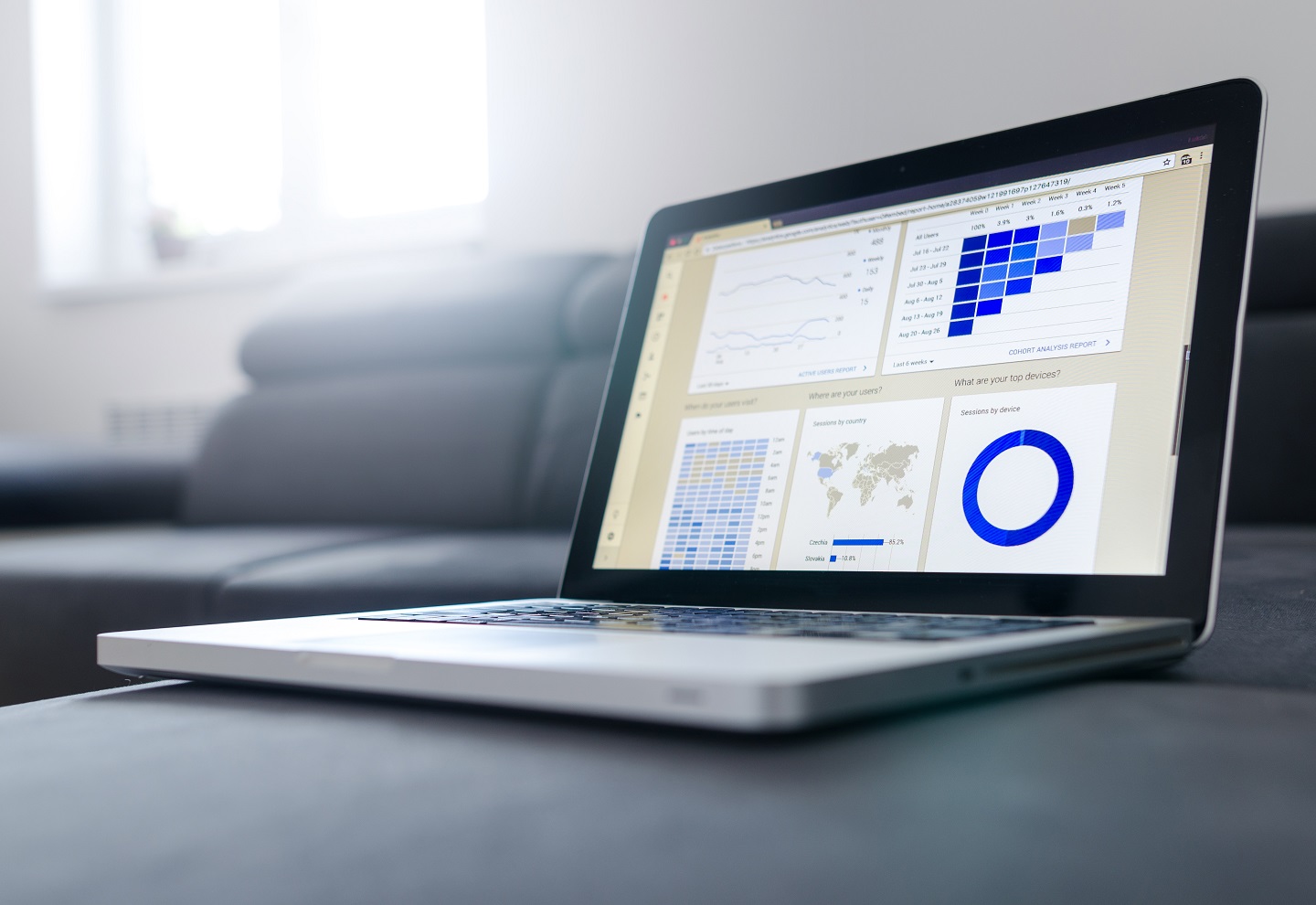
Smart data, not big data, can unlock the potential of predictive maintenance
Predictive Maintenance (PM) brings together and represents the potential of Industry 4.0. By combining sensors, connectivity and machine learning, PM helps anticipate equipment failures before they happen. This prevents over-maintenance and unnecessary stoppages, resulting in better equipment uptimes, improved quality and lower maintenance costs. The appeal of PM as the ‘killer app’ of Industry 4.0 is in its broad applicability in almost any sector, its intuitive benefits and lastly in its significant, measurable results. A recent estimate found that PM could improve asset availability by up to 15% and reduce maintenance costs by up to 25%. Those sorts of improvements get noticed.
PM comes with a sweet spot, however, where conditions enable it to add the most value. PM requires relevant and acquirable data to feed the machine learning, the data and potential failure sources should be of moderate volume to allow for meaningful results, and the economic stakes have to be high enough to warrant the invest of time and capital.
Be smart, not big
PM is also an illustrative example of how success in exploiting Industry 4.0 technologies is about going smart, not going big, especially when it comes to data.
One of the most appealing targets for PM are windmill generators. They fit nicely into the PM sweet spot of available performance and environmental data in moderate volumes, with huge economic costs to equipment downtime. A McKinsey study found that superior maintenance management may improve the IRR of a windfarm invest by up to 19%, and new projects have a lifetime expectancy of 30 years, double that of the 1990s.
With such compelling stakes, we spoke to Yvan Jacquat, CEO of Optimized Systems Technologies (OST), a firm specialized in providing turnkey PM solutions from sensors to analytics, including windmills for utilities customers, about the challenges of successfully implementing a windfarm PM program.
He pointed out some aspects of windfarms that make them particularly attractive candidates for PM. “Windfarms are usually in remote locations. This creates a challenge for availability of spare parts and personnel, and cranes for lifting of spare parts. Weather can be factor also, making smart and proactive maintenance planning critical.” Yvan also explained that windfarms are not like, say, production equipment in their capacity usage. “A production line must run to produce to demand. Any downtime will likely be made up through overtime. But windfarms can and should run every hour of every day. An hour lost to downtime is an hour that can never be recouped.”
Yvan explained that the first step is to be smart about data used for the PM analysis. “Customers often do not yet have a clear vision on what problems they are looking to address and imagine PM as a solution for everything. It’s easy to get lost in the weeds and try to solve every single maintenance or downtime issue. The key is not trying to capture and process every variable and try to solve every issue. We have to be smart about what are the critical failure modes to be detected proactively, what data is needed to support detection, what is the gap between the available and the required data and how to fill the gap at the lowest cost.”
OST has found that a smart combination of a handful of data points delivers much better PM than a pure analytics approach processing large sets of data. For example, production power data, processed with a few temperature readings through unsupervised machine learning, are able to predict fairly accurately power converter overheating leading to failures. This is very important for offshore wind farms, where data accessibility and connectivity are challenging dimensions.
This approach keeps data capture costs limited while still harvesting important PM returns quickly. This simplicity and turnaround offer other benefits as well. “There is a mindset in tension among managers when it comes to machine learning and analytics,” explained Yvan. “At the same time, they feel that it can solve all of their problems and also that it cannot reveal insights that they do not already possess. There is an apprehension of ‘black box’ solutions.” If the customer does not understand how an underlying problem has been uncovered and addressed, there can be resistance. By keeping the projects targeted, the solutions are clear enough that the customer can accept them, integrate them and leverage them.
Going Beyond PM
If the PM program has smartly chosen the data that will enable maintenance anticipation, the signal in the noise can offer other benefits. For windmills, one of these benefits was efficiency in power conversion. Yvan described how “During the course of our projects, we found underlying issues that had impacts beyond PM and were affecting the operational efficiency of the windmills in generating electricity. Issues on the pitch, yaw or generator can easily affect the power output by 3%. Our AI tool helps to detect such issues at the right time and quantify their impact on the power efficiency.”
By bringing these sorts of tangible benefits and ROI, PM can be way to leverage data, connectivity and AI to address real-world problems, alleviate managerial reluctance, and change the conversation from big data to smart data.
Ralf Seifert is Professor of Operations Management at IMD. He directs IMD’s new Digital Supply Chain Management program, which addresses both traditional supply chain strategy and implementation issues as well as digitalization trends and new technologies.
Richard Markoff is a supply chain researcher, consultant, coach and lecturer. He has worked in supply chain for L’Oréal for 22 years, in Canada, the US and France, spanning the entire value chain from manufacturing to customer collaboration.
Research Information & Knowledge Hub for additional information on IMD publications
This paper introduces the New Industrial Policy Observatory (NIPO) data set and documents emergent patterns of policy intervention during 2023 asso...
The case is seen through the eyes of the newly appointed supply chain director at a cosmetics company based in Berlin. The general manager has task...
Few Business to Business (B2B) marketplaces have succeeded. Metalshub has successfully combined a software platform as a service, with a marketplac...
Data cooperatives, entities that allow individuals to pool together their personal data to gain collective bargaining power and enable them to mon...
There are three immediate challenges for companies that want to train fine-tuned AI models: 1) they require extensive, high-quality data — a scarce...
in The World Economy July 2024, vol. 47, no. 7, pp. 2762-2788, https://doi.org/10.1111/twec.13608
Research Information & Knowledge Hub for additional information on IMD publications
Published by International Institute for Management Development ©2024
Research Information & Knowledge Hub for additional information on IMD publications
Research Information & Knowledge Hub for additional information on IMD publications
Case reference: IMD-7-2457 ©2024
Research Information & Knowledge Hub for additional information on IMD publications
Research Information & Knowledge Hub for additional information on IMD publications
Research Information & Knowledge Hub for additional information on IMD publications
Research Information & Knowledge Hub for additional information on IMD publications
in I by IMD Magazine March 2024, no. 13, pp. 26-28
Research Information & Knowledge Hub for additional information on IMD publications
Research Information & Knowledge Hub for additional information on IMD publications
Research Information & Knowledge Hub for additional information on IMD publications