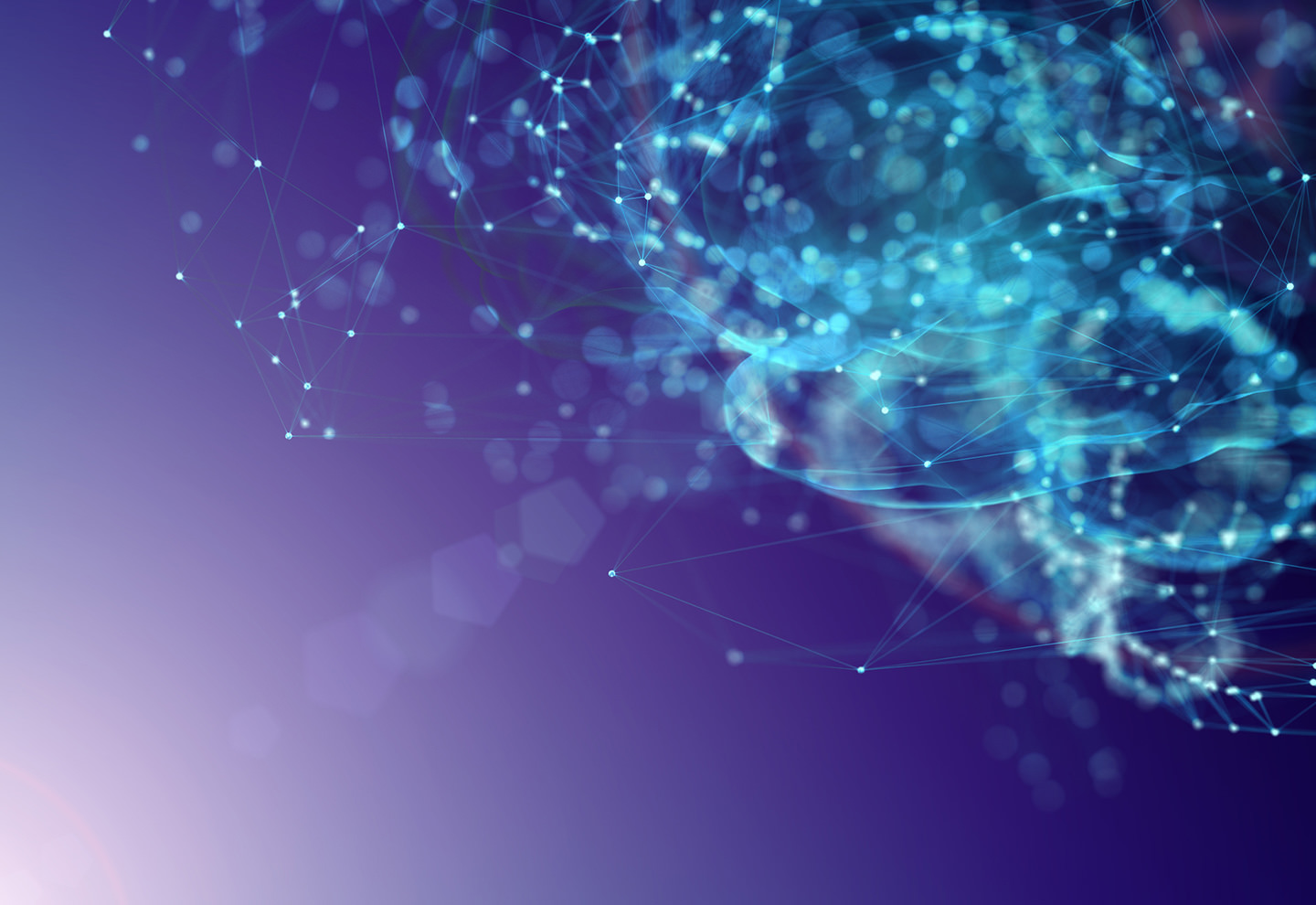
Artificial intelligence: one area where startups can teach established firms
During the last few years, immense hype has built up around artificial intelligence (AI) and machine learning. Thought leaders and industry captains alike have been hailing them as inventions that will transform humanity. As Andrew Ng, founder of the Google Brain Project and of Coursera famously stated, “AI is the new electricity.”
For organizations around the world, the scramble to join the AI race requires them to turn research conducted in labs into actual applications. And it is at this point that many have come to a grinding halt.
Our interactions with executives of established industrial giants reveal that (a) they are facing difficulties that are varied in nature, when trying to turn their inventions into viable products and services (b) they have a thing or two to learn in this area from young startups.
Our insights were the result of visits to numerous startups, technology giants and research universities across North America, Europe and Asia. Here, we share the five biggest learnings:
1- Don’t use AI if simpler techniques will work
A recurring message, especially from the most innovative startups (such as Gong.io in Tel Aviv) was that old-fashioned data analytics and visualization can still create value for organizations. When analyzing sales calls, for instance, Gong found that one of the most valuable functionalities of older, simpler techniques was tracking how long the salesperson was speaking. A pop-up or nudge if a monologue went on for too long was as effective as any cutting-edge sentiment analysis.
All too often, large organizations fall into the trap of overengineering their solutions and using complex technical methods where simpler ones will do. Startups have no such luxury. As a result, startups often come up with low-tech, but effective, solutions for major problems.
2- In a rush towards the artificial, don’t forget the human
Human intelligence that is. AI has demonstrated some amazing capabilities in the lab, matching humans at several soft skills such as mood and sentiment analysis, negotiation, collaboration and teamwork. Foundations such as the Allen Institute for AI are trying to teach AI common sense, while several other researchers are training AI to be ethical. Stories about these advances often blind organizations to the (im)practicalities of implementing these techniques.
Zebra Medical, a world leader in processing medical images, markets its core product as an inexpensive second opinion. They are not intended to nor are they capable of replacing humans. Managers would do well to maintain humans at the forefront of decision-making, with support from other techniques. When asked how we could train AI to think just like humans, the leading AI scientist and thinker Moshe BenBassat memorably said, “Why would you want to?” Rather, he preferred “graceful interventions” to guide humans toward better decisions.
3- Data, data, data
We asked the most innovative startups what their competitive advantage was, and the verdict was loud and clear: having access to the right data. Whether it was worker-level data from shop floors of large manufacturers, sensor data from large machines, highly curated and accurately labeled medical data or real-world driving data, founders were forthright in admitting that most of their techniques were based on fairly generic algorithms.
The reason that they were so highly valued was that they had allowed these algorithms to learn from unique datasets that were not available to even large, established competitors. Given that most firms are sitting on vast datasets that are not being leveraged, it makes sense to begin by applying simpler techniques to in-house data, rather than diving headfirst into creating complex algorithms.
4- Turns out ethics is an economic problem
Before Amnon Shashua could scale up Mobileye and sell it to Intel for USD $16 billion, he had to struggle with issues of liability (among others). His system alerted drivers if their vehicle was straying from their lane, whilst also providing other driver-assistance systems, which may eventually become the foundation of autonomous driving technology. Questions arose such as: Who is to blame if the system fails? And, how could they test the system and report on its reliability?
Shashua eventually realized that these were economic problems. They could collect massive amounts of data to prove reliability, but that would imply billions of miles of driving—given the low rates at which accidents occur. Alternatively, they could define hard rules that the system had to follow, like how to react in ‘right of way’ situations. However, these rules are never foolproof, and cannot account for all conditions.
His solution? Finding a happy medium that limited what the Mobileye system could do while at the same time maintaining its usefulness for drivers, and simultaneously satisfying regulators. As a startup, it was crucial for Mobileye to demonstrate financial sustainability, while being compliant with regulations and holding ethical standards. These entrepreneurs eventually broke their problem down into an economic one, arriving at a satisfactory rather than perfect solution.
5- It takes a village
Not only did these startups fascinate us, but also the ecosystem in which they were allowed to flourish. In Silicon Valley, it was the influx of money following AI ideas, access to top human capital and spillovers from locally based tech giants. In Israel it was government incentives for the Startup Nation, military applications and the famous ‘chutzpah’ culture. Across the world, founders we spoke to agreed that there was no single factor that could drive success in this area. While this is true for any kind of startup, it is especially so for AI babies.
Mooly Eden, former president of Intel Israel, told us that the cost of AI startups was actually cheaper than other types of startups. The real reason they flourished in some places more than others was the ecosystem. Traditional firms need to recreate these ecosystems internally. Simply supporting incubators or buying startups is unlikely to provide the impetus to innovate in the AI space.
The decade of 2010-2020 has seen AI advance by leaps and bounds. The next decade (2020-2030) promises to be one of commercializing these advances. Large organizations must be intelligent, seize the day and learn from small startups on how to do so now.
This article is part of a series on digital innovation in Israel.
Research Information & Knowledge Hub for additional information on IMD publications
Assortment optimization presents a complex challenge for retailers, as it depends on numerous decision factors. Changes in assortment can result in demand redistribution with multi-layered consequences. This complexity is even more pronounced for ...
in European Journal of Operational Research August 2025, vol. 324, no. 3, pp. 799-813, https://doi.org/10.1016/j.ejor.2025.01.035
Research Information & Knowledge Hub for additional information on IMD publications
Research Information & Knowledge Hub for additional information on IMD publications
in I by IMD
Research Information & Knowledge Hub for additional information on IMD publications
in I by IMD
Research Information & Knowledge Hub for additional information on IMD publications
in I by IMD
Research Information & Knowledge Hub for additional information on IMD publications
in I by IMD
Research Information & Knowledge Hub for additional information on IMD publications
Research Information & Knowledge Hub for additional information on IMD publications
in I by IMD
Research Information & Knowledge Hub for additional information on IMD publications
in I by IMD
Research Information & Knowledge Hub for additional information on IMD publications
Research Information & Knowledge Hub for additional information on IMD publications