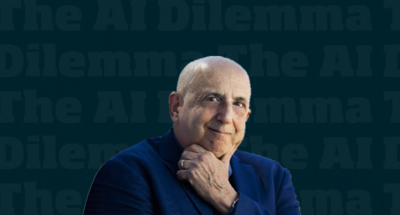
The AI Dilemma: If we are as gods, we may as well get good at itÂ
AI holds incredible promise to improve virtually every aspect of our lives, but we can't ignore its risks, mishaps, and misuses, author Art Kleiner tells José Parra Moyano....
by Tomoko Yokoi Published 10 April 2024 in Sustainability • 7 min read
While the hype around generative artificial intelligence (GenAI) shows little sign of abating, an undercurrent of negativity also appears to be gathering pace. Concerns around GenAI range from anxiety about high-profile hallucinations to complaints that the large language models (LLMs) it requires are built on the back of others’ work through plagiaristic training. And now the clamour is also growing about the environmental impact of GenAI – and artificial intelligence more broadly.
Take research cited in a February edition of Nature. It reveals how the tech giants, including Microsoft, Google and Meta, have dramatically increased their water consumption as they have needed to cool their growing numbers of data centres. And it warns AI demand will drive up these companies’ water withdrawal – when water is taken from the ground or surface sources – to as much as 6.6bn cubic metres by 2027. That’s roughly equivalent to half the total annual water consumption of the UK.
Given water scarcity issues in so many parts of the world, the analysis is worrying, but this paper is just the latest evidence of the technology sector’s growing environmental impact. An industry that once looked refreshingly green and clean compared to industrial businesses increasingly feels anything but. GenAI will only add to such worries, given the computational power it requires and the energy this consumes. As long ago as 2019, one study put the carbon footprint of training a single early LLM such as GPT-2 at about 300,000kg of carbon dioxide emissions – the equivalent of 125 round-trip flights between New York and Beijing. Since then, LLMs – and, by extension, their training footprints – have only got larger.Moreover, the training of LLMs is only the beginning. Once the model is put to work, responding to each new inquiry requires additional energy – maybe only a tiny amount in individual cases, but ChatGPT alone now gets 10 million requests every day.
Estimating the scale of that toll is not easy, but a recent project made progress and pointed out that generative computing architectures consume significantly more energy than task-specific systems
Let’s be fair. Technology companies’ total contribution to climate change remains small compared to other industries. In 2020, estimates for the information and communications technologies sector’s share of global greenhouse gas emissions ranged from 1.8% to 2.8%. The power sector, by contrast, accounts for a much as 38% of emissions.
It’s also worth saying that applications of AI include climate change mitigation, with the World Economic Forum arguing that the technology has the potential to play a huge role. Nevertheless, it is increasingly clear that the technology sector has a problem. Just as developers must address other concerns about GenAI, so must reducing its environmental impact become a priority. Otherwise, greenhouse gas emissions will increase markedly just at a time when other industries are pursuing decarbonization. Issues such as water scarcity cannot simply be brushed aside.
The first challenge in this regard will be to improve monitoring and measurement of AI’s carbon footprint. Without an accurate baseline and the ability to measure emissions accurately on an ongoing basis, there will be little confidence in the sector’s efforts – and claims – on reduction strategies.
This is not straightforward. For one thing, while many technology companies are working hard to measure their emissions, most seem reluctant to provide any data on what proportion of their energy usage is accounted for by AI. That makes it very difficult to hold them to account on their environmental performance specifically relating to AI. It won’t be easy to work out how quickly the growth of AI is driving demand for energy, for example, or to establish whether mitigation efforts in this area are paying dividends.
A broader problem has been the lack of consensus about how exactly to measure the energy consumption of AI. Some models focus on energy usage measurements made iteratively while others attempt to aggregate use; attempts to align usage data to information such as local emissions are similarly inconsistent.
The good news is that we are beginning to see improvements in this area, with several tools and initiatives designed to make it easier to determine a reliable emissions baseline. The Green Software Foundation’s Software Carbon Intensity specification is one good example. Elsewhere, tools such as the Green Algorithms calculator provide a means with which to estimate the carbon footprints of specific projects – including AI initiatives and high-performance computing. Stanford University researchers have published a framework for more reliable, straightforward and accurate reporting of energy usage in machine learning systems.
We are also seeing the industry beginning to acknowledge the problem. Amazon Web Services, Google Cloud Platform and Microsoft Azure all now make carbon accounting tools available that are specific to their products and services. These at least provide standardised measures for different projects and initiatives run on the same cloud service.
“While many technology companies are working hard to measure their emissions, most seem reluctant to provide any data on what proportion of their energy usage is accounted for by AI.”
Still, measuring greenhouse gas emissions is only the first hurdle to clear. Industry leaders will also want to take practical but definite steps towards mitigating their climate change impacts – and be seen to be doing so. That will require them to address AI-related emissions at each stage of the lifecycle of every project, from development and training through to deployment and usage.
There are multiple opportunities to explore. By some estimates, switching to renewable energy grids for the training of LLMs could reduce the emissions at this stage of an AI initiative by a factor of 40. There is also a need for more efficient GPUs, as well as the possibility of buying carbon credits for emissions that can’t easily be avoided.
There is also scope to reduce the amount of energy that a given AI project actually requires. Computationally efficient algorithms will produce dividends, for example. It should become standard practice for developers to focus on training time and other relevant parameters as they assess the performance of new initiatives.
For maximum effect, it will be necessary to combine multiple strategies. A recent paper from Google and the University of California, Berkeley, recommends an approach centred on the “four Ms” – model, machine, mechanisation and mapping. It advocates for more refined software algorithms, more energy efficient computer hardware, greater energy efficiency in the data centre too, and better location of data centres for access to cleaner energy.
There is a role for policymakers here too – with approaches that focus on both carrot and stick. The former might include more use of tax incentives for the development of data centres in locations with good access to hydro and solar power. The latter might include tougher regulation on disclosure – airlines required to make data available that enables the analysis of emissions from individual flights, for example, may wonder why the technology sector is able to hide behind aggregated numbers.
Get all of this right and it is possible to make a significant difference. The Google-UC Berkeley paper points to the launch of the Generalist Language Model system launched a few months after the higher-profile debut of GPT-3. By optimizing the four Ms, the GlaM project reduced carbon emissions by a factor of 14. The same paper suggests improvements each of the four Ms over four years to 2021 meant the average data centre opened that year would have consumed so much less energy that it would have produced 747 times fewer carbon emissions than the average facility in 2017.
Will this be sufficient to mitigate surging demand for GenAI applications – and to counter the growing criticism of the climate change impacts of this rapidly evolving technology? It represents a beginning. Responsible GenAI businesses will build on these foundations as new opportunities for mitigation emerge – but they must make a start now.
Researcher, Global Center for Digital Business Transformation, IMD
Tomoko Yokoi is an IMD researcher and senior business executive with expertise in digital business transformations, women in tech, and digital innovation. With 20 years of experience in B2B and B2C industries, her insights are regularly published in outlets such as Forbes and MIT Sloan Management Review.
26 April 2024 • by José Parra Moyano in Artificial intelligence
AI holds incredible promise to improve virtually every aspect of our lives, but we can't ignore its risks, mishaps, and misuses, author Art Kleiner tells José Parra Moyano....
23 April 2024 • by Jerry Davis in Artificial intelligence
GenAI can deliver cost and productivity benefits for colleges and students, but there is a dark side....
22 April 2024 • by José Parra Moyano, Christine Legner, Konrad Schulte in Artificial intelligence
Training and fine-tuning AI models requires troves of data, but storing and processing that data is financially and environmentally costly. Here are three ways to address the problem....
18 April 2024 • by Quentin Gallea in Artificial intelligence
GenAI will allow human talent to focus on more creative, complex tasks, leading to greater job satisfaction and well-being....
Explore first person business intelligence from top minds curated for a global executive audience