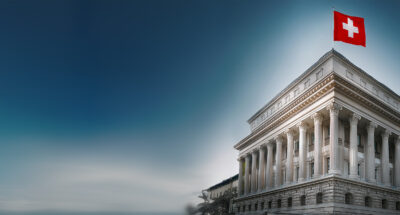
Swiss banking: In search of a secure future
In light of the Swiss Federal government’s report following the Credit Suisse debacle, how can the Swiss banking sector regain its reputation for stability and resilience? ...
by Jon Danielsson, Andreas Uthemann Published 8 March 2024 in Finance • 6 min read
The rapidly growing use of AI promises much-improved efficiency in the delivery of financial services – but it will also create new threats to financial stability that arise when AI vulnerabilities interact with frailties in the financial system.
AI is now widely applied to such tasks as risk management, asset allocation, credit decisions, fraud detection, and regulatory compliance. The financial authorities already employ AI for low-level analysis and forecasting. They are expected to expand its use to designing, monitoring, and enforcing financial regulations, identifying and mitigating financial instabilities, and resolving failing institutions and crises.
Building on previous work in this field, we identified four sources, or “channels,” where the use of AI could pose societal risks.
Malice is a particular concern in the financial system because it is full of highly resourced, profit-maximizing economic operators, many of whom are unconcerned about the social consequences of their activities. These users can bypass controls and change the system by manipulating AI engines or using them to find loopholes to evade control. They may even deliberately create market stress, which can be highly profitable for those forewarned.
We expect the most common malicious use of AI will be by employees of financial institutions, who are careful to stay on the right side of the law. AI will likely also facilitate illegal activities, such as rogue traders and criminals, as well as terrorists and nation-states aiming to create social disorder.
The second area of concern arises when AI users are misinformed about its abilities but are also strongly dependent on it. This is most likely to happen when data-driven algorithms, such as those used by AI, are asked to extrapolate to areas where data is scarce and objectives unclear, which is very common in the financial system.
AI engines are designed to provide advice even when they have very low confidence about the accuracy of their answer. They can make up facts or present arguments that sound plausible but would be considered flawed or incorrect by an expert – both instances of the broader phenomenon of “AI hallucination.” The risk here is that the AI engines will present confident recommendations about outcomes they know little about.
“While concerns about how AI can destabilise the financial system might make us careful in adopting AI, we suspect it will not.”
The third channel of instability emerges from the difficulties in aligning the objectives of AI with those of its human operators. AI is much better at handling complexity than humans, and while it can be instructed to behave like a human, there is no guarantee it will do so, and it is almost impossible to pre-specify all the objectives AI has to meet. This is a problem, since AI is very good at manipulating markets and is not concerned with the ethical and legal consequences of its actions unless explicitly instructed.
Research has shown how AI can spontaneously choose to violate the law in its pursuit of profit. Using GPT-4 to analyze stock trading, the AI engine was told that insider trading was unacceptable; however, when the engine was given an illegal stock tip, it proceeded to trade on it and lie to the human overseers: an example, perhaps, of AI mirroring the illegal behavior of humans.
The superior performance of AI can also destabilize the system even when it is only doing what it is supposed to do. More generally, AI will find it easy to evade oversight because it is very difficult to patrol an almost infinitely complex financial system. AI can keep the system stable while aiding the forces of instability at the same time. In its complexity, it is always one step ahead of humans: the more we use AI, the more complex the computational problem for the authorities becomes.
The final risk area emerges from the business model of those companies designing and running AI engines. AI analytics businesses depend on three scarce resources: computers with the requisite GPUs, human capital, and data. An enterprise that controls the biggest share of each will likely occupy a dominant position in the financial AI analytics business. As a result, the AI industry is being pushed towards an oligopolistic market structure dominated by a few large vendors. The end result is uniformity across the sector and an absence of the competitive edge that might detect systemic fragilities earlier.
As the oligopolistic nature of the AI analytics business increases systemic financial risk, it is cause for concern that neither the competition nor the financial authorities appear to have fully appreciated the potential for increased systemic risk due to oligopolistic AI technology in the recent wave of data-vendor mergers.
The expanding use of AI in the private and public sectors presents enormous efficiency gains and cost-saving advantages. While concerns about how AI could destabilize the financial system might make us careful in adopting it, we suspect it will not. Technology is often initially met with skepticism, but as it becomes apparent that it is out-performing what came before, it is increasingly trusted.
Nonetheless, we should be careful not to over-focus on these issues. The benefit of AI will likely be overwhelmingly positive in the financial system – as long as the authorities are alive to the threats and adapt regulations to meet them. The ultimate risk is that AI becomes irreplaceable and a source of systemic risk before the authorities have formulated an appropriate response.
This is an edited version of the VoxEU article How AI can undermine financial stability. Any opinions and conclusions expressed here are those of the authors and do not necessarily represent the views of the Bank of Canada.
Director, Systemic Risk Centre at LSE
Jon Danielsson is director of Systemic Risk Centre and Reader of Finance at the London School of Economics. He has also worked for the Bank of Japan and the International Monetary Fund. Since receiving his PhD in the economics of financial markets from Duke University in 1991, Jón’s work has focused on how economic policy can lead to prosperity or disaster. He is an authority on both the technical aspects of risk forecasting and the optimal policies that governments and regulators should pursue in this area.
Principal Researcher at the Bank of Canada.
Andreas Uthemann is a principal researcher at the Bank of Canada. He is a research associate of the LSE’s Systemic Risk Centre and a research affiliate of the Centre for Finance at UCL. His research is in financial economics with a focus on market structure and design, financial intermediation, and financial regulation. He obtained a PhD in Economics from University College London.
19 July 2024 • by Peter Nathanial, Ludo Van der Heyden, Salvatore Cantale in Finance
In light of the Swiss Federal government’s report following the Credit Suisse debacle, how can the Swiss banking sector regain its reputation for stability and resilience? ...
17 July 2024 • by Michael R. Wade, Evangelos Syrigos in Finance
Companies that excel in both digital and sustainable transformation attract a stock market premium, according to research. So, how do you tap into that value? ...
10 July 2024 • by Michael Skapinker in Finance
A rise in climate change protests against leading arts institutions spells trouble for the future of corporate sponsorship. Companies and cultural organizations must learn to manage the risks....
8 July 2024 • by Richard Baldwin in Finance
As the world’s largest democracy prepares to overtake China in terms of economic growth, it offers a huge investment opportunity, explains IMD’s Richard Baldwin....
Explore first person business intelligence from top minds curated for a global executive audience