IMD business school for management and leadership courses
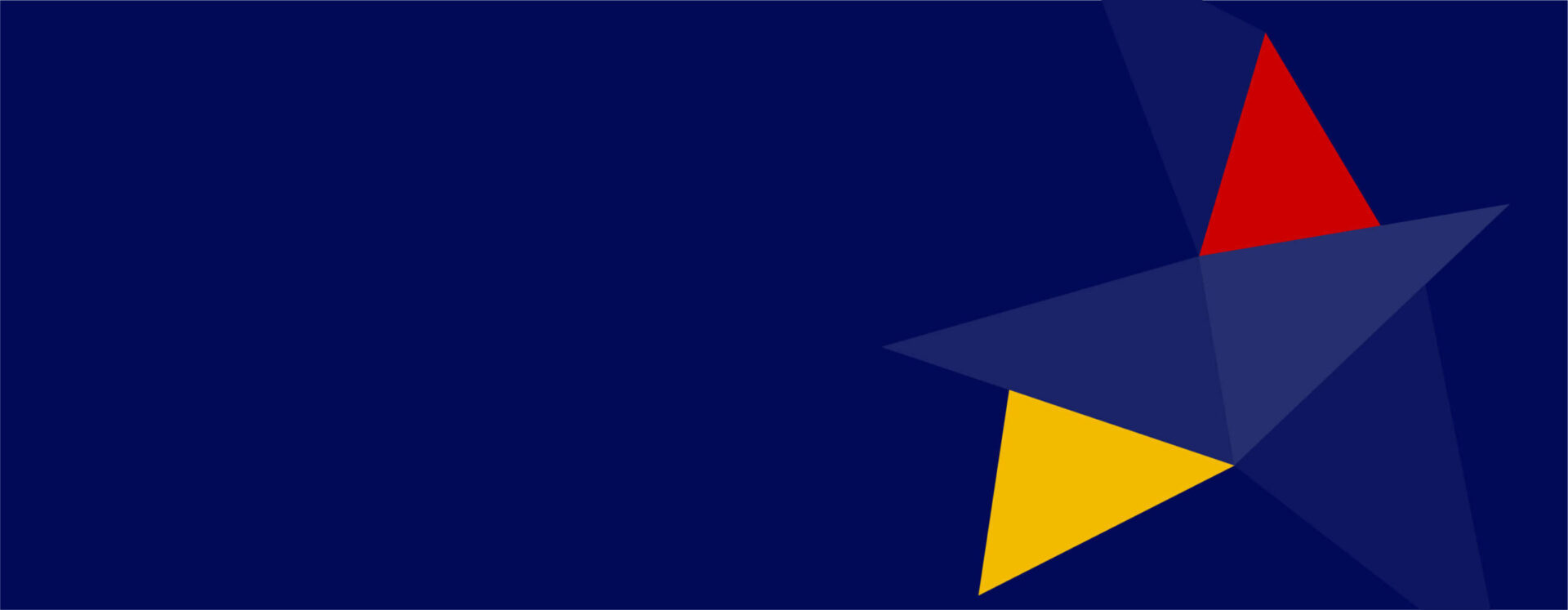
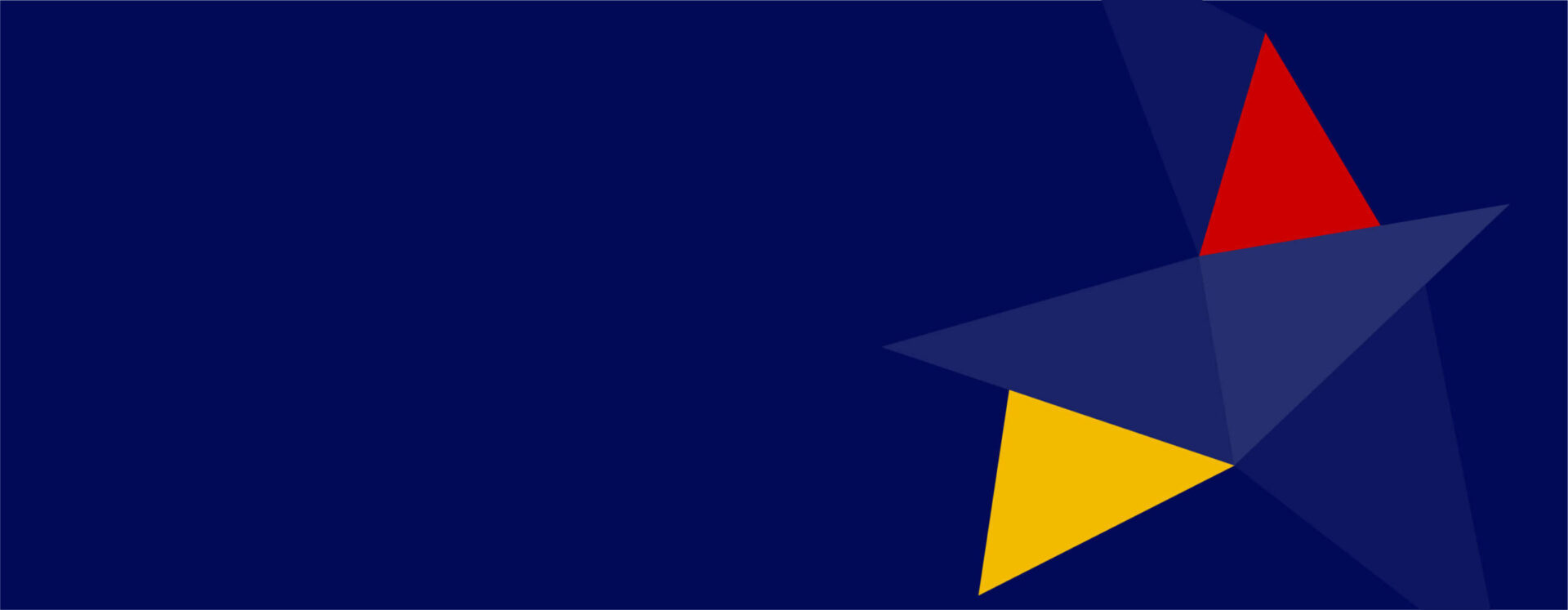
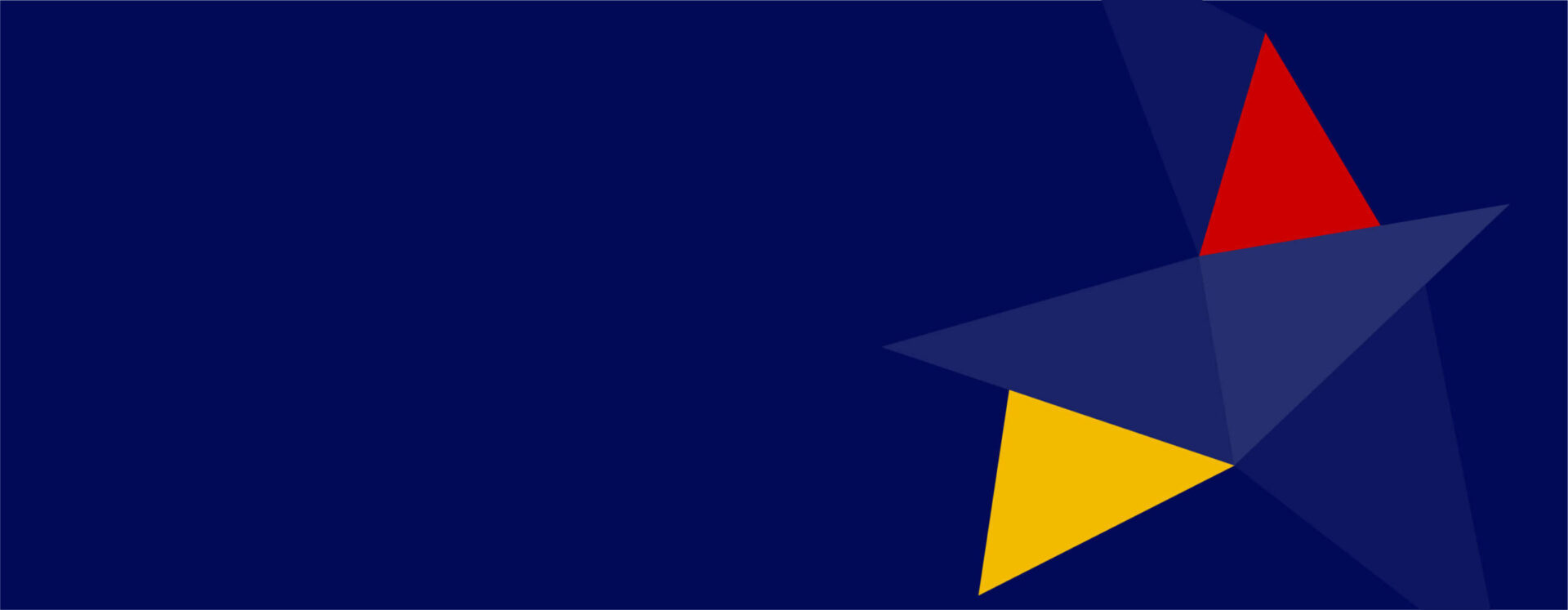
A.I. Text Analysis
How does artificial intelligence help us understand companies better than ever before?
Artificial intelligence (AI) enables us to gain deeper insights into companies by analyzing textual information, uncovering patterns in language style, and capturing nuanced discussions around specific topics. AI-driven methodologies allow us to explore not just what executives or media say about companies, but how they say it, and with what intensity. Specifically, we use advanced AI techniques to perform two distinct types of analysis—style-based analysis and subject-based analysis— leveraging textual data from two primary sources: over 70 major news sources and earnings calls transcripts.
We employ two specialized AI algorithms designed for these analyses: Augmented co-occurence and Textual Entailment.
Augmented Co-Occurence
Augmented Co-Occurence builds upon traditional linguistic analysis methods such as the Linguistic Inquiry and Word Count (LIWC) framework.
Specifically, Augmented Co-Occurence employs predefined dictionaries consisting of individual words, sequences of words, or pattern-based expressions (similar to regular expressions) derived from peer-reviewed academic specifications. These dictionaries capture psychological, linguistic, and strategic constructs based on their relative frequencies within texts.
However, unlike traditional LIWC, our Augmented Co-Occurence incorporates advanced NLP techniques including:
- Coreference Resolution: Utilizing transformer-based models to accurately replace pronouns and ambiguous references with explicit company names.
- Negation Detection: Identifying and interpreting negations within text, ensuring precise measurement of constructs (for example, distinguishing between a positive trait like “fast-moving” and a negative formulation such as “not fast-moving”).
Textual Entailment
Textual Entailment leverages a sophisticated Natural Language Processing (NLP) technique that evaluates whether a given piece of text logically supports predefined hypotheses. By analyzing textual data at the sentence level, this method allows us to explore both the linguistic style used by executives (through earnings call transcripts) and the subjects discussed in both executive communications and external news articles, along with their intensity.
Specifically, we define precise hypotheses aligned with distinct strategic and personality traits, as summarized in Fact Sheet below.
By computing sentence-level entailment probabilities, we aggregate these results into company-level metrics that quantify the intensity of each style (in executive transcripts) or subject (in both executive transcripts and news articles). This approach facilitates nuanced comparisons across companies, highlighting differences in leadership style based on internal executive communications and highlights strategic focus based on both internal and external textual sources.
By computing sentence-level entailment probabilities, we aggregate these results into company-level metrics that quantify the intensity each style or subject. This allows for nuanced comparisons of company style of leadership as well as company focus.