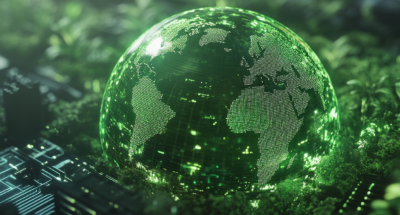
Are you using AI for a sustainable future?
As AI continues to reshape industries, businesses must navigate the balance between automation and human contribution. Take this short quiz to test your knowledge of its potential uses and check out our...
Published May 14, 2025 in Brain Circuits • 2 min read
While DeepSeek is fast and less expensive than its US competitors, independent security evaluations have found a series of weaknesses in it.
“By proactively integrating security measures into AI development and deployment, organizations can minimize risks.”
May 7, 2025 • by José Parra Moyano in AI
As AI continues to reshape industries, businesses must navigate the balance between automation and human contribution. Take this short quiz to test your knowledge of its potential uses and check out our...
April 17, 2025 • by Alexander Fleischmann in AI
A recent survey by IMD, Microsoft, and Ringier reveals that just 35% of organizations have strategies to address diversity bias in GenAI. Alexander Fleischmann identifies the risks and suggests ways to mitigate...
April 10, 2025 • by Sarena Lin , Tania Lennon in AI
Expertise and skills are becoming redundant faster than ever. But why is expertise needed in the AI age? Test your understanding and see our tips on developing cutting-edge expertise as the lifecycle of skills shrinks....
April 9, 2025 • by Tomoko Yokoi in AI
Deep tech is often described as a “first-of-a-kind” innovation built on cutting-edge research, such as new materials, synthetic biology, fusion energy, and quantum computing. Unlike consumer products or digital offerings, deep tech...